Getting Started¶
This notebook gets you started with a brief nDCG evaluation with LensKit for Python.
Setup¶
We first import the LensKit components we need:
[1]:
from lenskit import batch, topn, util
from lenskit import crossfold as xf
from lenskit.algorithms import als, item_knn as knn
from lenskit.metrics import topn as tnmetrics
And Pandas is very useful:
[2]:
import pandas as pd
[3]:
%matplotlib inline
Loading Data¶
We’re going to use the ML-100K data set:
[4]:
ratings = pd.read_csv('ml-100k/u.data', sep='\t',
names=['user', 'item', 'rating', 'timestamp'])
ratings.head()
[4]:
user | item | rating | timestamp | |
---|---|---|---|---|
0 | 196 | 242 | 3 | 881250949 |
1 | 186 | 302 | 3 | 891717742 |
2 | 22 | 377 | 1 | 878887116 |
3 | 244 | 51 | 2 | 880606923 |
4 | 166 | 346 | 1 | 886397596 |
Defining Algorithms¶
Let’s set up two algorithms:
[5]:
algo_ii = knn.ItemItem(20)
algo_als = als.BiasedMF(50)
Running the Evaluation¶
In LensKit, our evaluation proceeds in 2 steps:
- Generate recommendations
- Measure them
If memory is a concern, we can measure while generating, but we will not do that for now.
We will first define a function to generate recommendations from one algorithm over a single partition of the data set. It will take an algorithm, a train set, and a test set, and return the recommendations.
Note: before fitting the algorithm, we clone it. Some algorithms misbehave when fit multiple times.
The code function looks like this:
[6]:
def eval(aname, algo, train, test):
fittable = util.clone(algo)
algo.fit(train)
users = test.user.unique()
# the recommend function can merge rating values
recs = batch.recommend(algo, users, 100,
topn.UnratedCandidates(train), test)
# add the algorithm
recs['Algorithm'] = aname
return recs
Now, we will loop over the data and the algorithms, and generate recommendations:
[7]:
all_recs = []
test_data = []
for train, test in xf.partition_users(ratings[['user', 'item', 'rating']], 5, xf.SampleFrac(0.2)):
test_data.append(test)
all_recs.append(eval('ItemItem', algo_ii, train, test))
all_recs.append(eval('ALS', algo_als, train, test))
With the results in place, we can concatenate them into a single data frame:
[8]:
all_recs = pd.concat(all_recs, ignore_index=True)
all_recs.head()
[8]:
item | score | user | rank | rating | Algorithm | |
---|---|---|---|---|---|---|
0 | 603 | 4.742555 | 6 | 1 | 0.0 | ItemItem |
1 | 357 | 4.556866 | 6 | 2 | 4.0 | ItemItem |
2 | 1398 | 4.493086 | 6 | 3 | 0.0 | ItemItem |
3 | 611 | 4.477099 | 6 | 4 | 0.0 | ItemItem |
4 | 1449 | 4.454879 | 6 | 5 | 0.0 | ItemItem |
[9]:
test_data = pd.concat(test_data, ignore_index=True)
nDCG is a per-user metric. Let’s compute it for each user.
However, there is a little nuance: the recommendation list does not contain the information needed to normalize the DCG. Specifically, the nDCG depends on all the user’s test items.
So we need to do three things:
- Compute DCG of the recommendation lists.
- Compute ideal DCGs for each test user
- Combine and compute normalized versions
We do assume here that each user only appears once per algorithm. Since our crossfold method partitions users, this is fine.
[10]:
user_dcg = all_recs.groupby(['Algorithm', 'user']).rating.apply(tnmetrics.dcg)
user_dcg = user_dcg.reset_index(name='DCG')
user_dcg.head()
[10]:
Algorithm | user | DCG | |
---|---|---|---|
0 | ALS | 1 | 11.556574 |
1 | ALS | 2 | 7.383188 |
2 | ALS | 3 | 1.223253 |
3 | ALS | 4 | 0.000000 |
4 | ALS | 5 | 4.857249 |
[11]:
ideal_dcg = tnmetrics.compute_ideal_dcgs(test)
ideal_dcg.head()
[11]:
user | ideal_dcg | |
---|---|---|
0 | 4 | 16.946678 |
1 | 14 | 34.937142 |
2 | 15 | 25.770188 |
3 | 22 | 34.698538 |
4 | 23 | 41.289861 |
[12]:
user_ndcg = pd.merge(user_dcg, ideal_dcg)
user_ndcg['nDCG'] = user_ndcg.DCG / user_ndcg.ideal_dcg
user_ndcg.head()
[12]:
Algorithm | user | DCG | ideal_dcg | nDCG | |
---|---|---|---|---|---|
0 | ALS | 4 | 0.000000 | 16.946678 | 0.000000 |
1 | ItemItem | 4 | 0.000000 | 16.946678 | 0.000000 |
2 | ALS | 14 | 7.060065 | 34.937142 | 0.202079 |
3 | ItemItem | 14 | 7.218123 | 34.937142 | 0.206603 |
4 | ALS | 15 | 1.773982 | 25.770188 | 0.068839 |
Now we have nDCG values!
[13]:
user_ndcg.groupby('Algorithm').nDCG.mean()
[13]:
Algorithm
ALS 0.133029
ItemItem 0.104659
Name: nDCG, dtype: float64
[14]:
user_ndcg.groupby('Algorithm').nDCG.mean().plot.bar()
[14]:
<matplotlib.axes._subplots.AxesSubplot at 0x2643b7a0cf8>
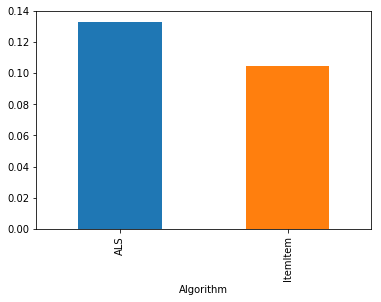
[ ]: